Our research group uses computational analysis and machine learning algorithms to identify patterns and relationships from complex, multimodal, and multiscale datasets of the brain in health and disease. We collaborate with national and international partners to apply novel methods from the fields of computational biology and computer vision to obtain new insights from brain datasets, with a primary focus on neurovascular and neurodegenerative phenotypes. We focus on learning interpretable representations from complex high-dimensional datasets that allow us to readily explain the biological relevance of results and ultimately understand underlying disease mechanisms.
We are particularly interested in the following areas:
1) Spatial Omics:
We aim to gain a deeper understanding of the cellular organisation of the brain by analysing gene and protein expression in a spatial context. Using spatial omics datasets, including 10x and MERFISH, we extract spatial gene and protein expression patterns and relate them to morphology and single-cell RNA sequencing data. For this, we have recently developed the spatial omics analysis toolbox squidpy (Palla, Spitzer, et al, Nature Methods 2021) and the subcellular analysis method campa (Spitzer, Berry et al, Nature Methods 2023, in press) in collaboration with Fabian Theis at Helmholtz Munich.
2) Image Representation Learning:
We establish pipelines for extracting interpretable features from histology and neuroimaging data using advanced deep learning algorithms. As part of the MELD project in collaboration with Sophie Adler and Konrad Wagstyl at University College London, we are currently using graph neural networks to identify focal cortical dysplasia lesions in a large cohort of epilepsy patients (Spitzer, Ripart et al, Brain 2022). Our goal is to develop and apply advanced image analysis techniques that will help us to better understand structural brain changes associated with neurodegenerative diseases.
3) Cross Modal and Cross Dataset Learning:
We aim to combine datasets across diseases and modalities into a multimodal and multi-scale brain atlas. By integrating data from different sources and diseases, we hope to identify features that are conserved across diseases and study correlations between modalities. This will enable us to better understand disease phenotypes and mechanisms which might lead to more effective treatment strategies.
Our lab is a part of the Institute of Stroke and Dementia Research, and we are affiliated with the Computational Health Center at Helmholtz Munich. We are currently looking for talented and motivated Masters students, PhD students and postdocs to join our team!
Please contact hannah.spitzer@med.uni-muenchen.de to learn more.
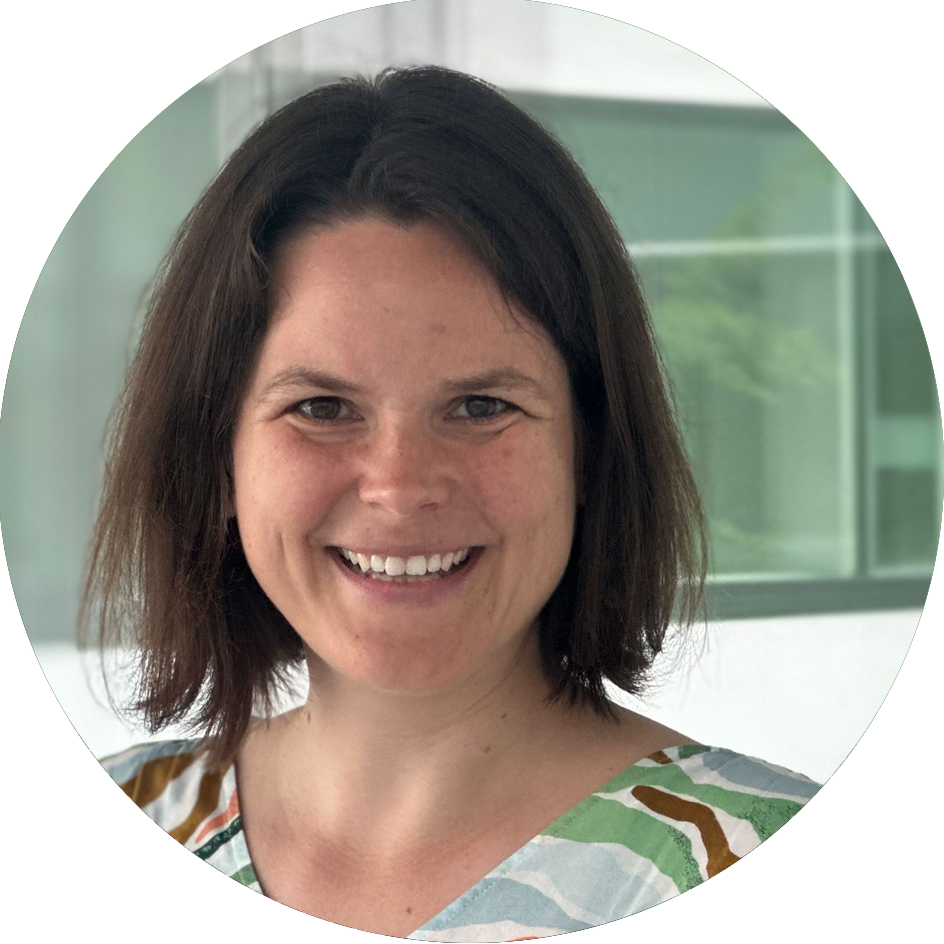
Hanna Spitzer, PI
see more about the PI on the next section
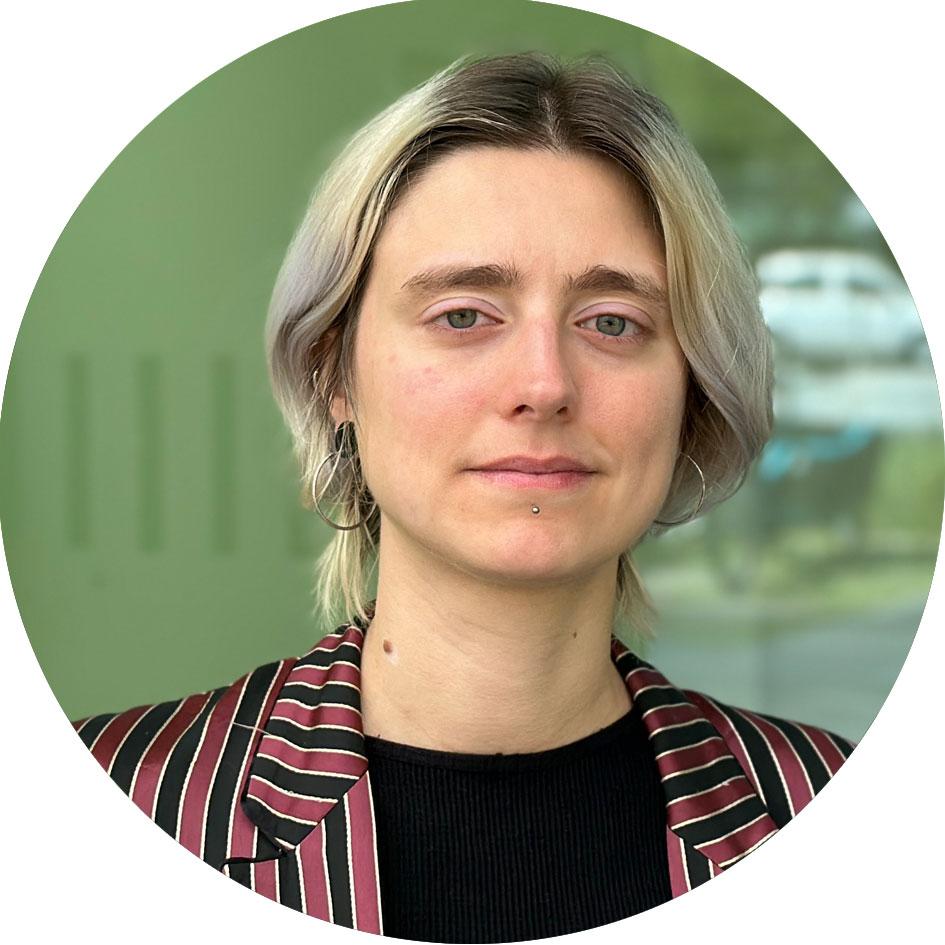
Beatrice Guastella, PhD Student
I completed my BSc studies in Pharmaceutical Biotechnology at the University of Milan in 2019, and my MSC studies in Bioinformatics and Systems Biology in Amsterdam in 2021. I have always been fascinated by the application of mathematics and data science to neuroscience. After a short research experience at Leiden University, I joined the Spitzer lab in September 2023. My projects are focused on developing ML approaches for the analysis of imaging and omics data, in the context of neurovascular diseases. I have many hobbies, including drawing, animation, videogames, and inline skating. I am also very passionate about data justice, which principles I aim at integrating in my own work.
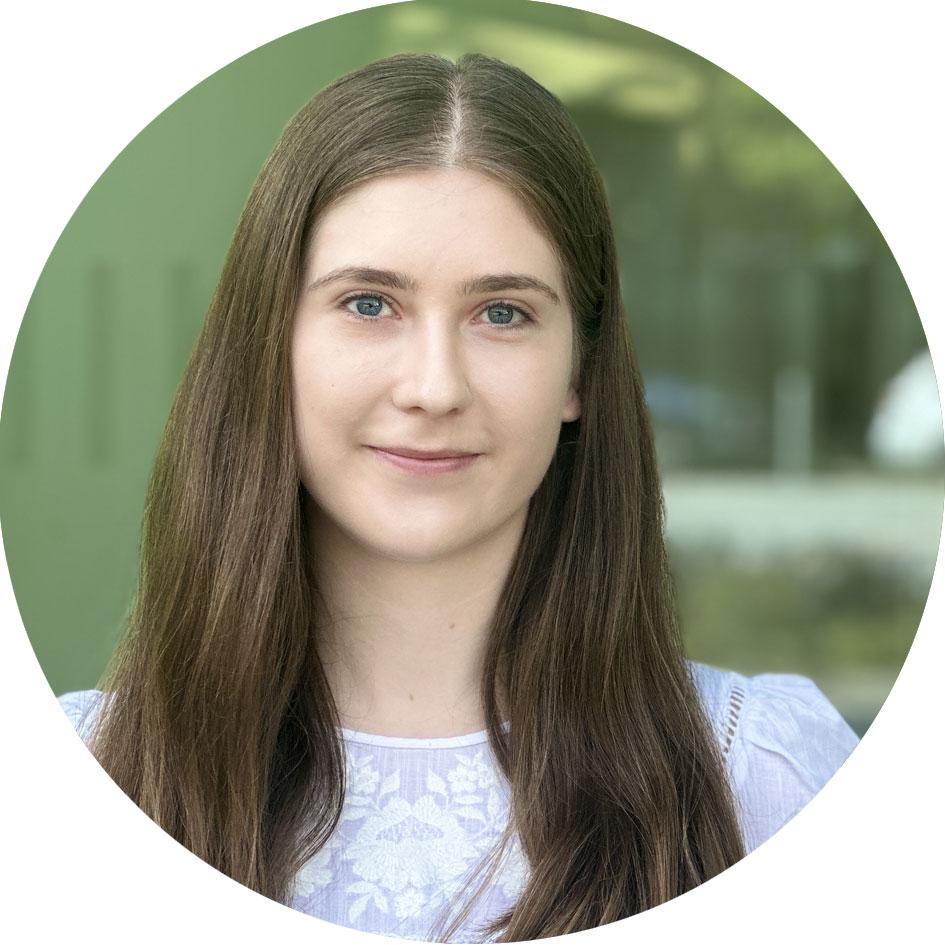
Katia Berr, PhD Student
I completed my bachelor's degree (2022) and master's degree (2024) in mathematics at the Technical University of Munich, where my master's thesis already focused on deep learning applications on brain data. After finishing my studies, I joined the Spitzer lab in July 2024 as a PhD student, specializing in representation learning of multimodal data, particularly medical images and spatial omics. Outside of my research, I enjoy reading fantasy novels, practicing gymnastics, and playing the violin.
Alumni:
Deniz Fettahoglu, MSc Student
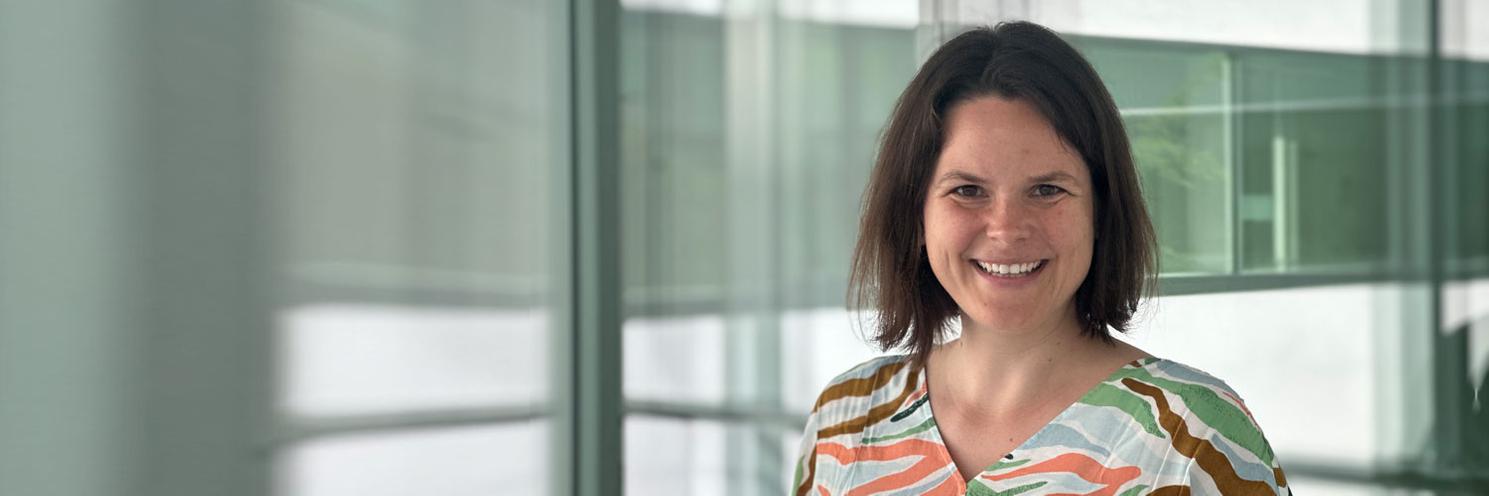
My research focus is on using machine learning algorithms to extract meaningful insights from biomedical image data and integrating them with molecular measurements. My work involves exploring supervised, self-supervised, and unsupervised approaches to tackle representation learning, classification, segmentation, and forecasting tasks in neuroscience, computational biology and medical applications. Close collaborations with biologists, medical researchers, and clinicians have enabled me to have a good understanding of the needs of these communities when it comes to usability and applicability of machine learning models.
In 2023 I joined the ISD to establish my own team focussing on integrating and analysing multimodal and multiscale brain datasets with advanced machine learning methods. The goal of my lab is to bring new advancements in data analysis and machine learning from computational biology and computer vision to neuroscientific data to advance our understanding of disease mechanisms.
Scientific career
2023 – now Research group leader, Institute of Stroke and Dementia Research, LMU, Germany & associated research group leader, Computational Health Center, Helmholtz Munich, Germany
2019 – 2023 Postdoctoral researcher, Institute of Computational Biology,
Helmholtz Munich, Germany, Prof F Theis
2019 visiting researcher at University College London, UK, Prof. J. Ashburner (2 months)
2018 visiting researcher at Montreal Neurological Institute, McGill University, Canada, Prof. A. Evans (1 month)
2015 – 2020 PhD in Computer Science, Prof. S. Harmeling and Prof K. Amunts, Heinrich-Heine University Düsseldorf, Germany and Research Center Jülich, Germany; “Automatic Analysis of Cortical Areas in Whole Brain Histological Sections using Convolutional Neural Networks”
2013 – 2015 Masters in Computer Science, RWTH Aachen, Germany
2009 – 2013 Bachelors in Computer Science, RWTH Aachen, Germany
2023
2022
Hannah Spitzer
Tel: +49-89-4400-46019
hannah.spitzer@med.uni-muenchen.de